Featured Projects
Boost high quality academic outputs
Sponsored by Chinese Academy of Sciences, there are 15 PhD students, postdocs and research assistants or associates will be trained via the joint research. More than 30 high quality research papers will be jointly published by the two parties, in top tier conferences and journals, producing significant impact in both academia and industry. The two parties will organize various forms of academic events, including forums, workshops and summer schools to gain international influences. This collaborative research will also provide an idea platform for the development of junior staff, where a few rising starts in AI, DS and Robotics are expected to be cultivated and to shine at international stages.
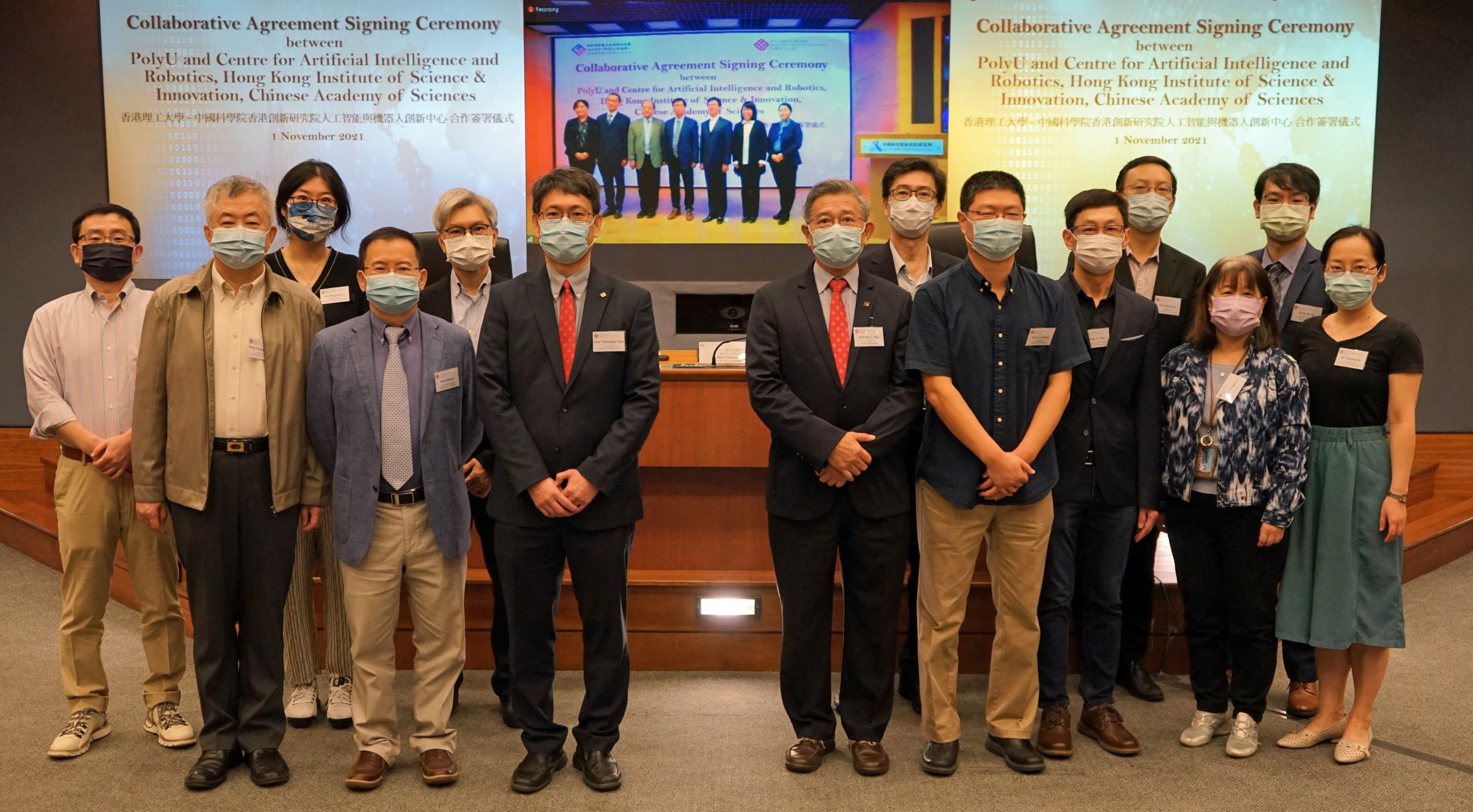
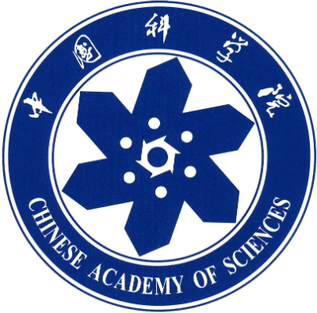
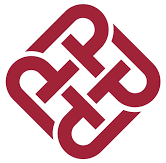
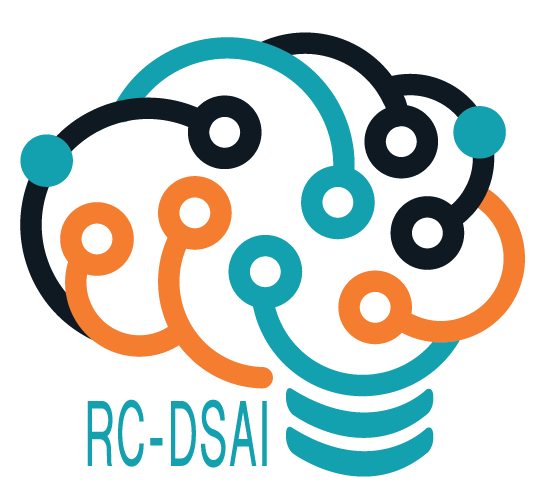
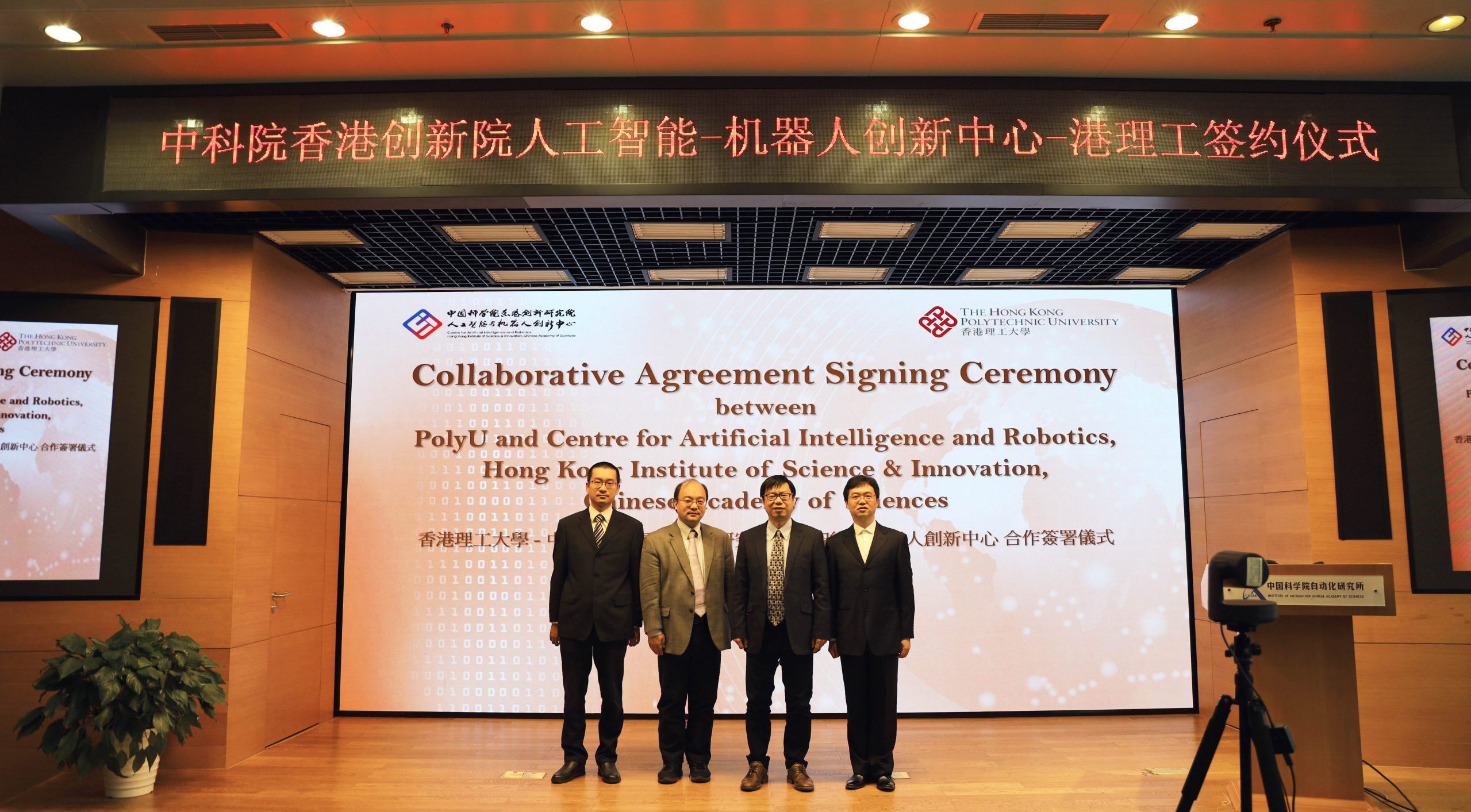
Solar Energy Performance Management System
This project develops a novel intelligent solar energy management system based AI, digital twin and big data analytics, which is a cloud-based holistic solar energy performance management platform for operational efficiency and performance enhancement for one or multiple PV systems locally, with integrated functions such as real-time monitoring, comprehensive system data collection, cross-system performance data sharing and benchmarking, advanced analytics for production prognosis and reliability analysis etc.
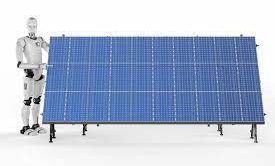
Short-term PVery Short-term Photovoltaic Power Forecasting Based on Cloud Tracking
The integration of PV power brings great economic and environmental benefits. However, the high penetration of PV power may challenge the planning and operation of the existing power system owing to the intermittence and randomicity of PV power generation. Achieving accurate forecasting for PV power generation is important for providing high quality electric energy for end-consumers and for enhancing the reliability of power system operation. Motivated by recent advancements in deep learning methods and their satisfactory performance in the energy sector, a hybrid deep learning model combining wavelet packet decomposition (WPD) and long short-term memory (LSTM) networks is proposed in this study. The hybrid deep learning model is utilized for one-hour-ahead PV power forecasting with five-minute intervals. WPD is first used to decompose the original PV power series into sub-series. Next, four independent LSTM networks are developed for these sub-series. Finally, the results predicted by each LSTM network are reconstructed and a linear weighting method is employed to obtain the final forecasting results. The performance of the proposed method is demonstrated with a case study using an actual dataset collected from Alice Springs, Australia. Comparisons with individual LSTM, recurrent neural network (RNN), gated recurrent (GRU), and multi-layer perceptron (MLP) models are also presented. The values of three performance evaluation indicators, MBE, MAPE, and RMSE, show that the proposed hybrid deep learning model exhibits superior performance in both forecasting accuracy and stability.
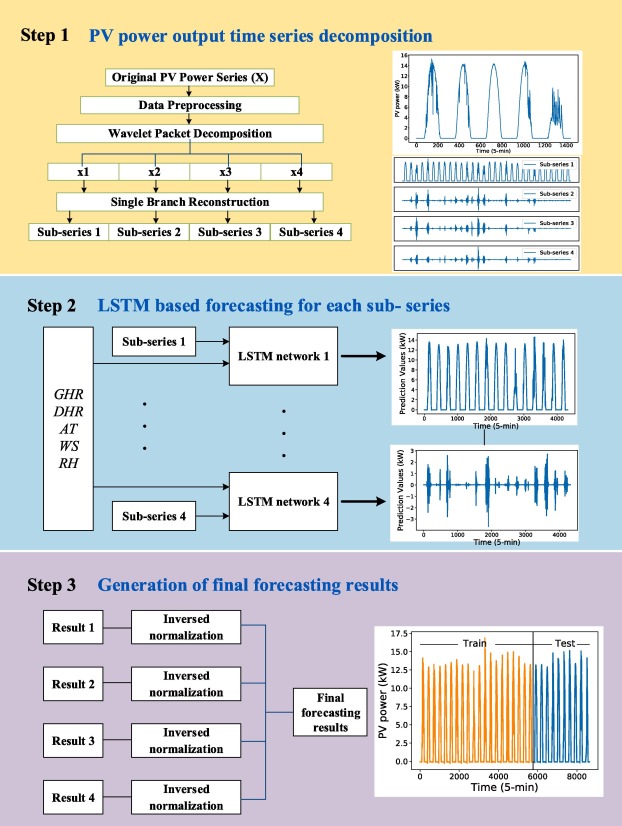
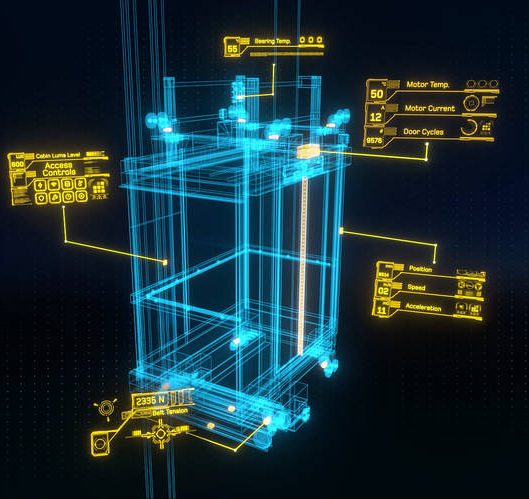
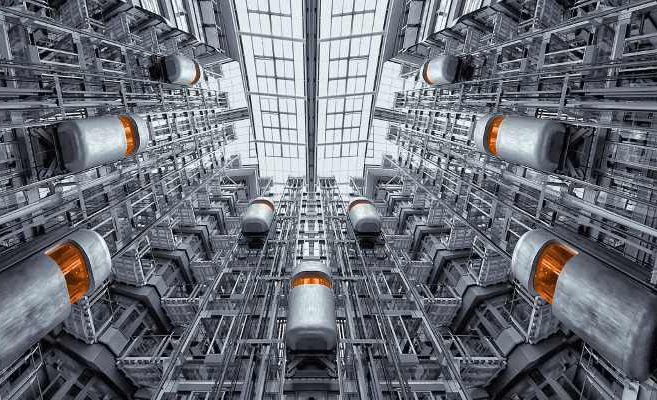
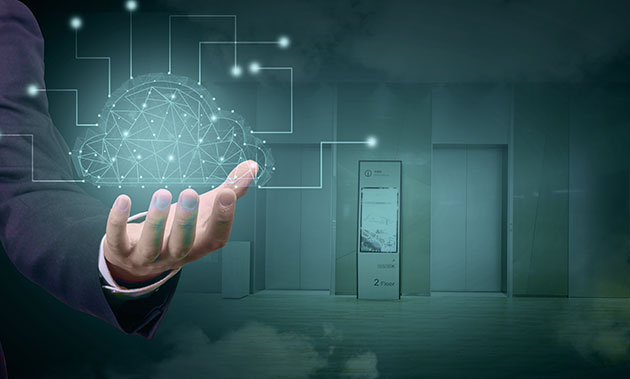
Machine Learning Based Adaptive Fault Prediction Data Analytics System for Lift Monitoring
Facility managers usually conduct reactive maintenance or preventive maintenance strategies in building maintenance management. However, there are some limitations that reactive maintenance cannot prevent failure, and preventive maintenance cannot predict the future condition of MEP components and repair in advance to extend the lifetime of facilities. Therefore, this study aims to apply a predictive maintenance strategy with advanced technologies to overcome these limitations. Building information modeling (BIM) and Internet of Things (IoT) have the potential to improve the efficiency of facility maintenance management (FMM). Despite the significant efforts that have been made to apply BIM and IoT to the architecture, engineering, construction, and facility management (AEC/FM) industry, BIM and IoT integration for FMM is still at an initial stage. In order to provide a better maintenance strategy for building facilities, a data-driven predictive maintenance planning framework based on BIM and IoT technologies for FMM was developed, consisting of an information layer and an application layer. Data collection and data integration among the BIM models, FM system, and IoT network are undertaken in the information layer, while the application layer contains four modules to achieve predictive maintenance, namely: (1) condition monitoring and fault alarming module, (2) condition assessment module, (3) condition prediction module, and (4) maintenance planning module. Machine learning algorithms, ANN and SVM, are used to predict the future condition of MEP components. Furthermore, the developed framework was applied in an illustrative example to validate the feasibility of the approach. The results show that the constantly updated data obtained from the information layer together with the machine learning algorithms in the application layer can efficiently predict the future condition of MEP components for maintenance planning.
Smart Space
The project aims to adopt latest research results in artificial intelligence (AI), data science (DS) and robotic technologies to create an environment where human beings can interact naturally with their electronic appliances such as coffee machines, washing machines and multimedia players etc. as similar to interact with another human.
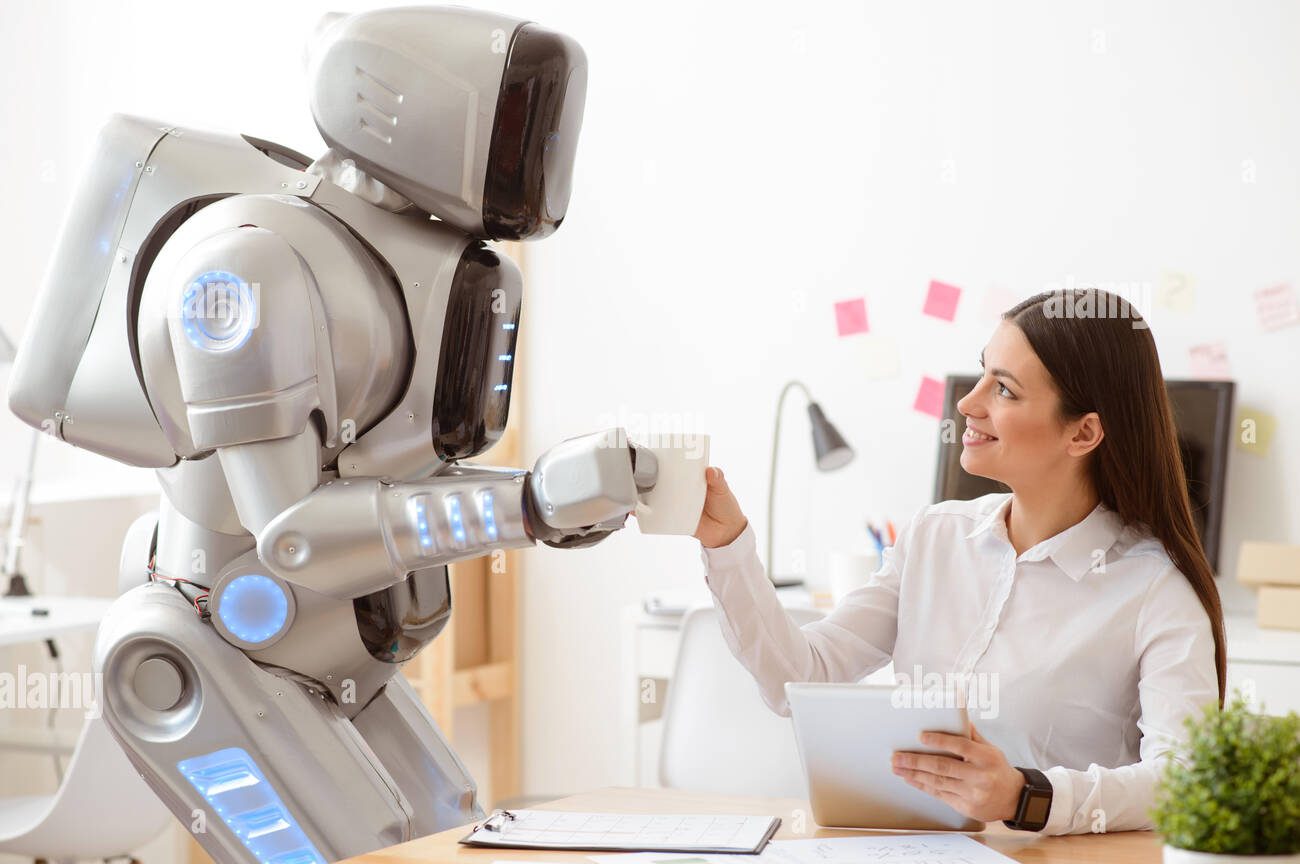
High Fidelity Solar Irradiance Forecasting for Solar Power Integration
Solar energy is an abundant and renewable alternative to fossil fuels with essential implication of carbon neutrality. If utilized efficiently, it has the potential to supply the energy demand of the entire globe. However, there are two major obstacles that hinder the rapid deployment of solar power systems: (1) high temporal and spatial variability of solar irradiance that would cause instability and failure of power girds; and (2) low power production density per unit land area that would cause land competitions with existing infrastructures. To achieve the net-zero emission goal by 2050 set by several countries, innovate designs for solar power systems are essential to overcome the abovementioned obstacles. Large-scale energy storage (e.g. batteries) could stabilize fluctuate solar power to the grid, but grid wide battery systems are still not economically viable. A low-cost alternative to decrease the uncertainty in the solar power output is forecasting the solar power production to help with real-time dispatch and grid balancing. Accurate forecasting will also benefit the real-time control of the storage system.
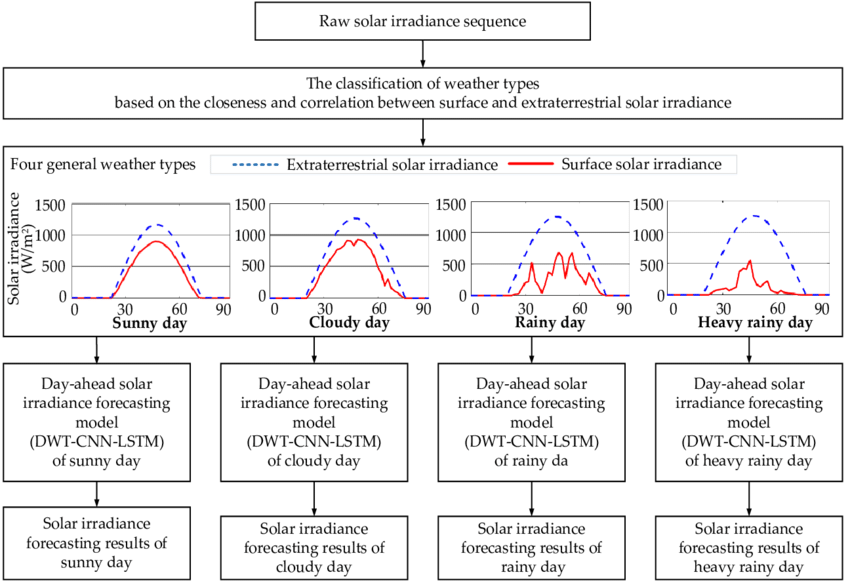
Development of Low-speed Unmanned Ground Vehicle in Campus Environment
There are four fundamental technical challenges to develop a low-speed unmanned ground vehicle (UGV); Localization, Perception, Control and Mapping. The AAE IPNL (Intelligent Positioning and Navigation Laboratory) has been working the state-of-the-art localization, mapping and control algorithm to realize this UGV. This UGV can potentially contribute to various applications including (but not limit to):
1. Automatic food/document delivery
2. Automatic cleaning in public areas such as parks.
3. Space and military applications.
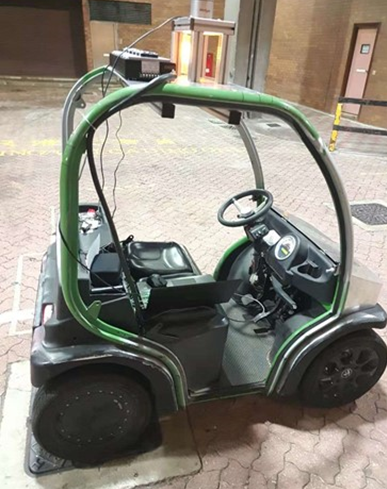
Mobile Exo-neuro-musculo-skeleton
The system is a light-weight mobile device with excellent rehabilitative effectiveness for tele-rehabilitation based on the internet of things. The invention of the exo-neuro-musculo-skeleton won a Grand Prize, a Gold Medal and a Special Merit Prize at the 47th International Exhibition of Inventions of Geneva in 2019. The invention was ranked as one of the Top 100 Science Spinoffs 2018, covering all industries in SPINOFF.COM Database (SDB) because of the uniqueness of the technology, high commercial potential and positive impact on the humanity’s well-being. The mobile exo-neuro-musculo-skeleton is also recognized as one of the five key developments which will impact the rehabilitation robotic global market from 2018 to 2026 by the COHERENT MARKET INSIGHTS.
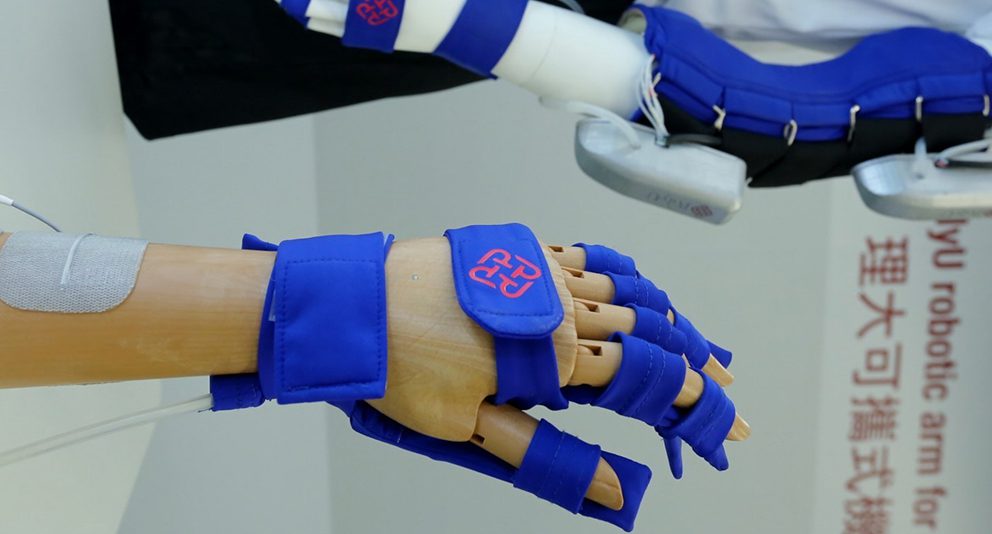
Cyber-Physical Asset Management System
There is an increasing trend to adopt indoor location asset tracking in enterprises to enable better supply chain visibility and more efficient operations. In the past, Radio Frequency IDentification technology (RFID), Bluetooth Low Energy (BLE) and Wifi are commonly used in indoor positioning systems. Recently, Ultra-wideband (UWB) is considered as the new ideal solution for asset management for complex environments. Comparing to other similar technologies, UWB performs better in different aspects including accuracy, cost efficiency, reliability, scalability and safety. In the future research direction, the UWB-based Cyber-Physical Asset Management System can locate and monitor the factory assets, equipment, workers and products in real time. It can also progressively conduct workflow and resources utilization analysis, and carry out predictive maintenance so as to optimize the operations and minimize the cost as well as the energy consumption
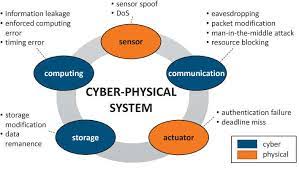
Intelligent workload balance control of the assembly process considering condition-based maintenancee
A fuzzy control system is developed to make real-time decisions to balance the workloads based on the processing abilities of workstations, given the policy of condition-based maintenance. Fuzzy controllers are used to decide whether to re-balance the assembly line and how to adjust the production rate of each workstation. It is showed that the buffer level of the assembly line with our fuzzy control system is lower than that of the assembly line without any control system and the buffer level of the assembly line with another control system is the lowest. The intelligent automation can improve the performance of the assembly process by the fuzzy control system since real-time information of the assembly line can be used for adaptive decision-making
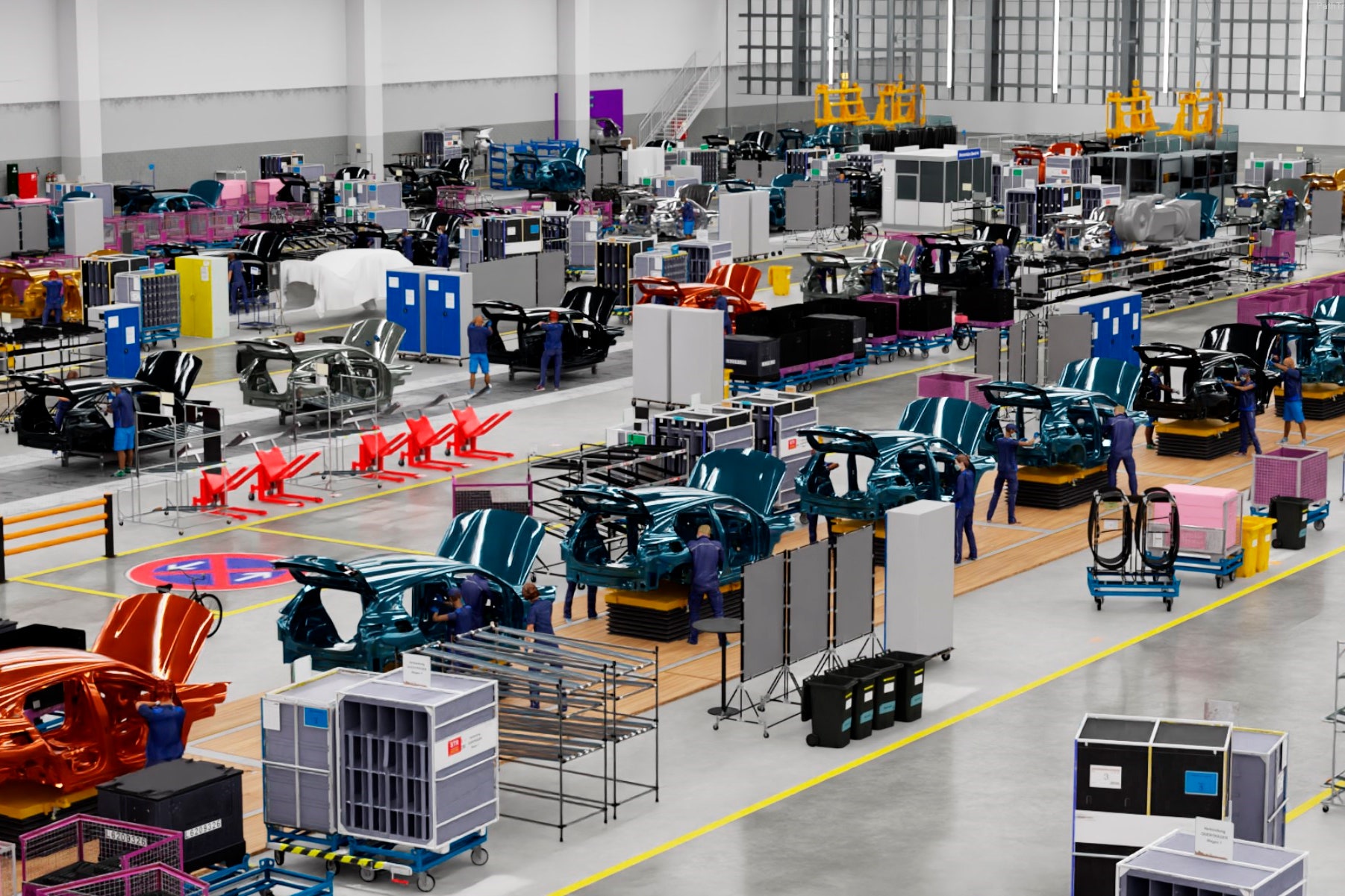